Autonomous Color Learning
This page describes the basic autonomous color learning scheme that works in our lab. In more recent results, we generalize the method to work in more uncontrolled conditions, such as indoor corridors. Recently, we have also combined this with our approach for illumination invariance to generate a method that enables the robot to autonomously detect and adapt to changes in illumination conditions.Color segmentation is a challenging subtask in computer vision. Most popular approaches are computationally expensive, involve an extensive off-line training phase and/or rely on a stationary camera. We present an approach for color learning on-board a legged robot with limited computational and memory resources. A key defining feature of the approach is that it works without any labeled training data. Rather it trains autonomously from a color-coded map of its environment. The process is fully implemented, completely autonomous, and provides high degree of segmentation accuracy.
Here, we present some image sequences that show the process that the robot goes through to learn the colors on the field. The videos are image sequences of that correspond to what the robot sees through its camera as it goes through the learning process. The sequences were generated by having the robot transmit the images to a PC, over the wireless network. Since the original sequences are several minutes long (see paper for details), we sampled them to select one frame for every six frames of the actual sequence. The sequences are therefore not at the actual frame rate (six times as fast). List of Image sequences (shown below):
-
Image sequence that shows the robot learning the marker colors in the
YCbCr color space.
-
Image sequence of the robot learning the marker colors in the LAB
color space.
-
Image sequence showing the robot learn the marker colors. Here each
frame consists of two images appended to each other. The image on the
left shows an external view of the robot performing the learning task
while that on the right (as before) shows the view of the world as
seen by the robot.
We also provide a set of images that show the segmentation results obtained by incorporating the color map learnt by the robot, using our approach. The images were captured using the robot's camera and segmented using the color maps learnt by the robot autonomously. Each set of images is suitably labeled by a caption. More details on the actual segmentation algorithm can be found in the paper.
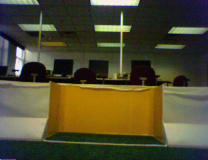
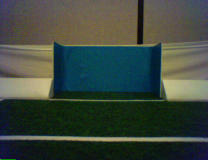

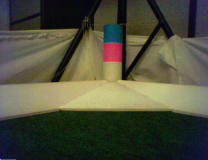
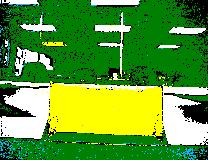
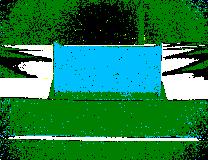
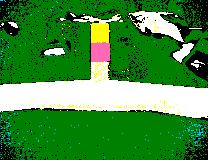
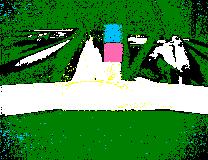
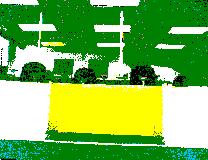
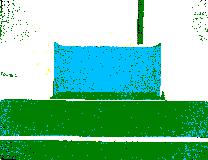
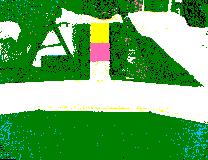
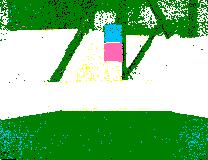
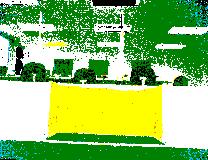
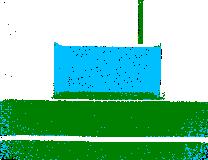
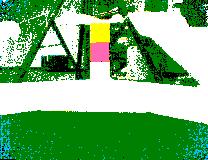
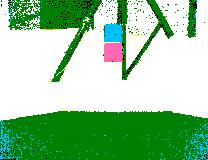
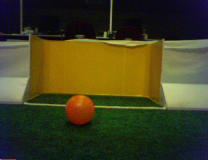
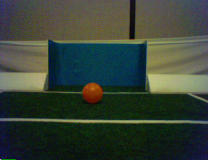
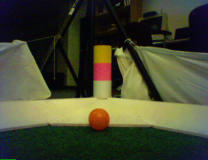
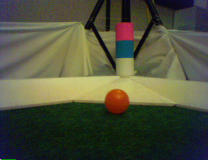
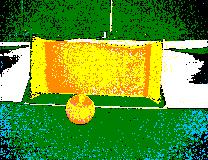
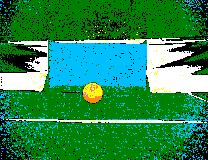

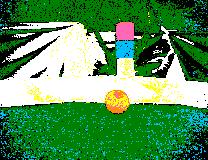
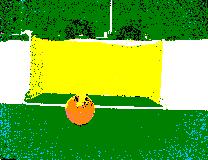

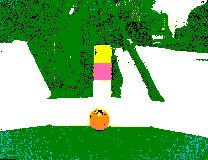
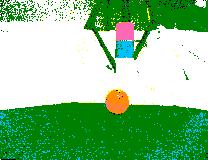
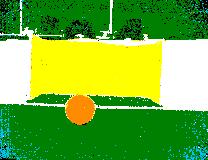
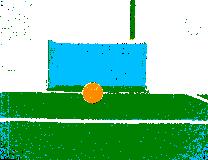
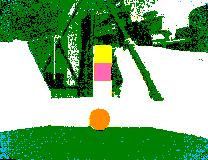
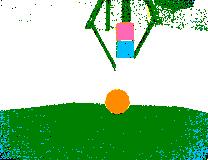
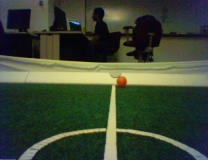
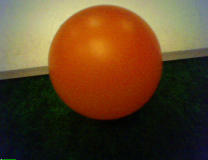
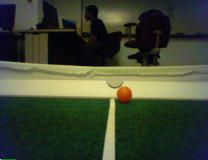
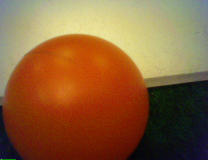
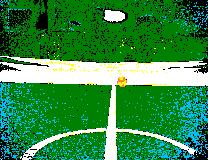
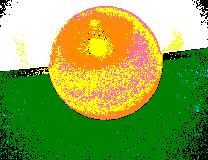
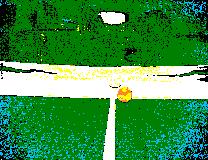
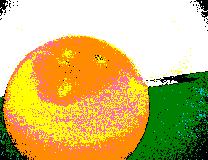
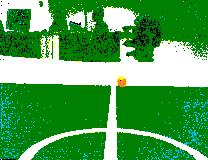
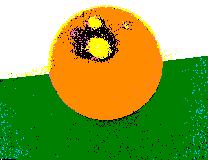
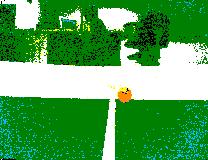
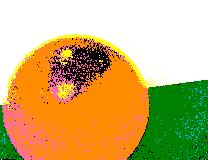
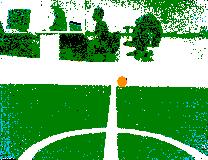
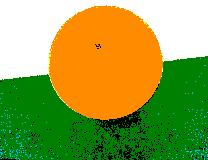
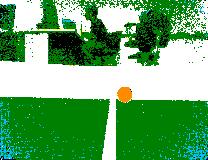
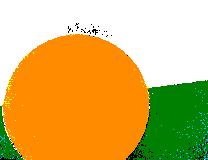
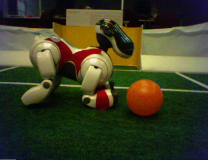
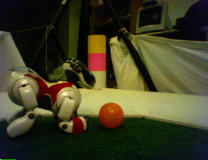
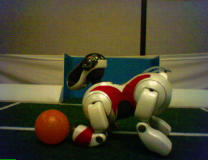
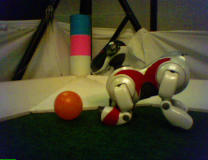
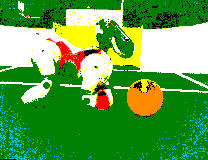
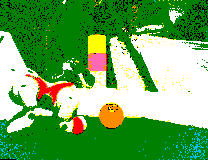
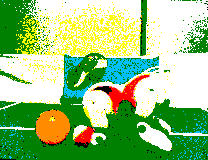
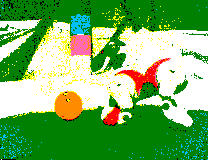
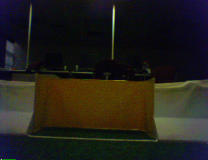
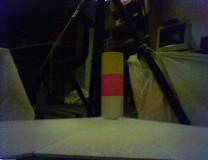
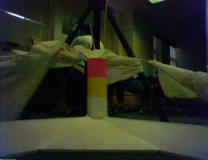
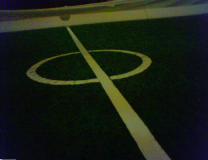
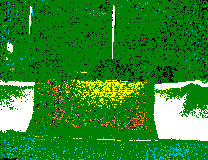
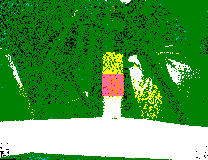
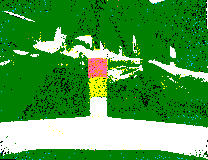
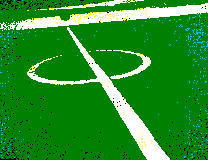
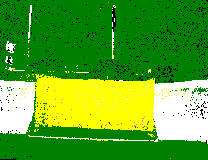
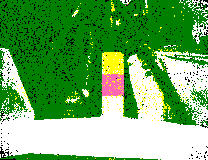
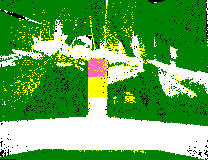
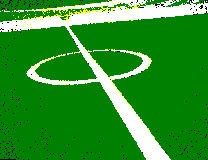
Full details of our approach are available in the following paper:
- Towards Eliminating Manual Color Calibration at RoboCup
Mohan Sridharan and Peter Stone.
RoboCup-2005: Robot Soccer World Cup IX, Springer Verlag, 2006.
- Modeling color using Gaussians works only within the lab setting and has problems when applied in more uncontrolled settings outside the lab.
- The motion sequence executed by the robot is provided by a human observer.