Learning 3D Foundation Models from Images
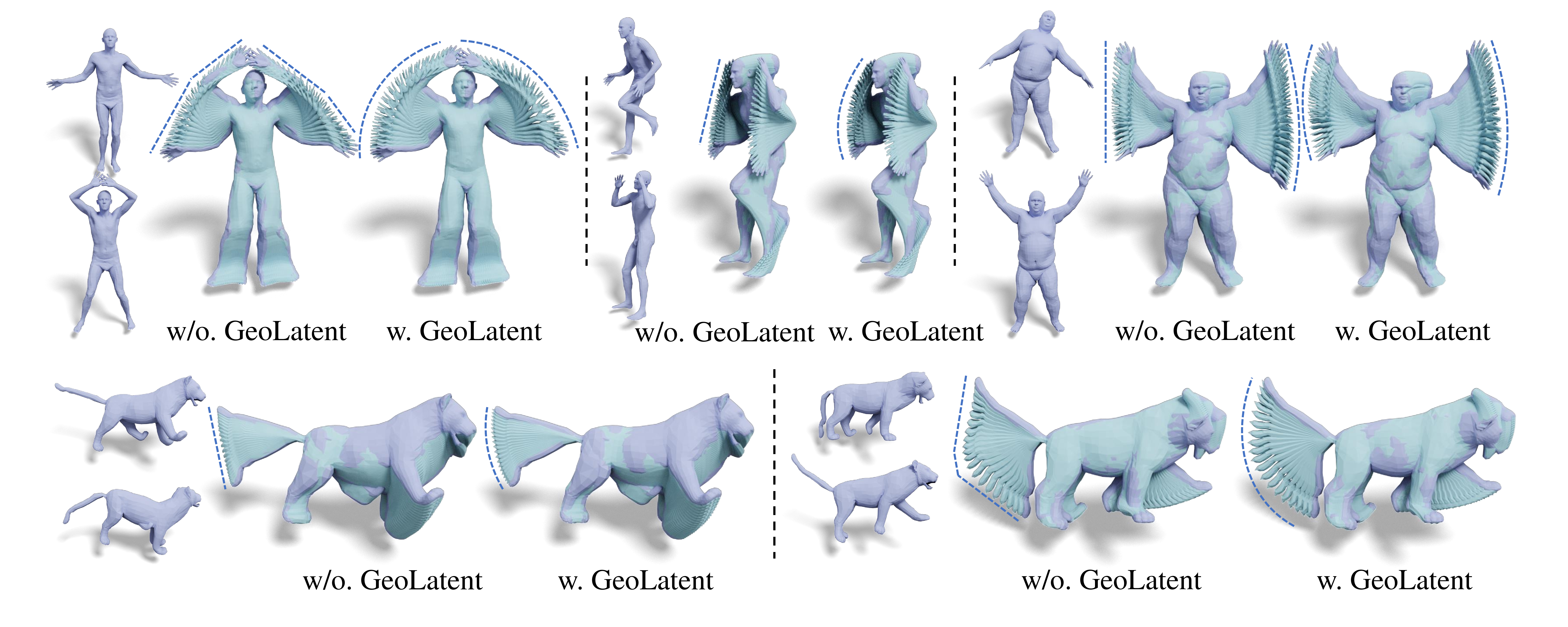
|
This is another central topic in my group. Foundation models have made remarkable progress in NLP and vision. A common message is that large-scale data and clean data are more important than the network architecture and training approach. The research on 3D deep learning has been tested on relatively small-scale dataset. A important question is do we still see big gaps under different 3D neural representations when having abundament 3D data? However, we do not and will not have large-scale 3D data as images. This brings the idea of learning 3D foundation models from images.
There are different aspects of this field. For example, people have observed that 2D foundation models reveal certain knowledge. When synthesizing different views of the same object, if the results are multi-view consistent, then we can obtain 3D objects. A recent paper studied this paper [Arxiv2024b]using pretrained video foundation models.
Another aspect is to perform neural rendering and train 3D foundation models from images. In a recent paper LEAP, we studied how to learn 3D representations from sparse-views where we do not have pose information. The paper was accepted by ICLR 2024.
Besides distilling 3D geometric information from image-based foundation models, my group is also interested in how to transfer texture information from images to 3D shapes. I started working on this topic in [SIGA16]. A recent paper [Arxiv2024a] studies this problem using pre-trained text-2-image models. The key task is to address multi-view consistency.
 Hanwen Jiang, Haitao Yang, Qixing Huang and Georgios Pavlakos. Real3D: Scaling Up Large Reconstruction Models with Real-World Images. https://arxiv.org/abs/2406.08479
|
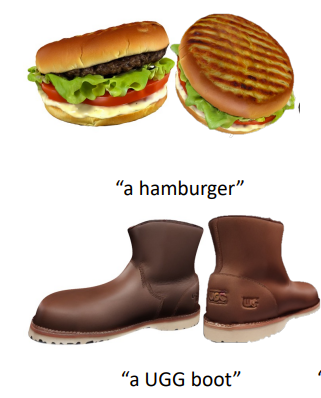 [Arxiv2024a] Zhengyi Zhao, Chen Song, Xiaodong Gu, Yuan Dong, Qi Zuo,Weihao Yuan, Zilong Dong, Liefeng Bo and Qixing Huang. An Optimization Framework to Enforce Multi-View Consistency for Texturing 3D Meshes Using Pre-Trained Text-to-Image Models. https://arxiv.org/abs/2403.15559
|
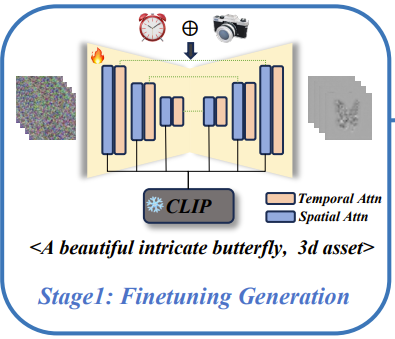 [Arxiv2024b] Qi Zuo, Xiaodong Gu, Lingteng Qiu, Yuan Dong, Zhengyi Zhao,Weihao Yuan, Rui Peng, Siyu Zhu, Zilong Dong,Liefeng Bo and Qixing Huang. VideoMV: Consistent Multi-View Generation Based on Large Video Generative Model. https://arxiv.org/abs/2403.12010
|
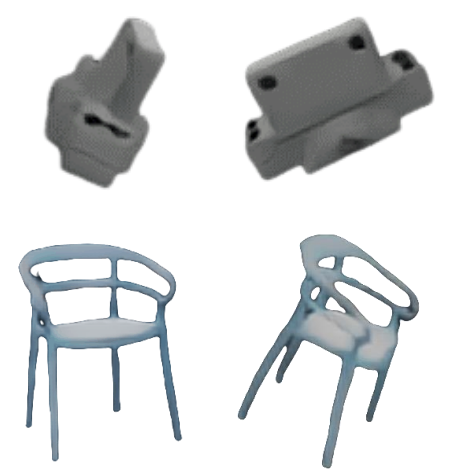 [ICLR24] Hanwen Jiang, Zhenyu Jiang, Yue Zhao and Qixing Huang. LEAP: Liberate Sparse-view 3D Modeling from Camera Poses. International Conferences on Learning Representations (ICLR) 2024
|
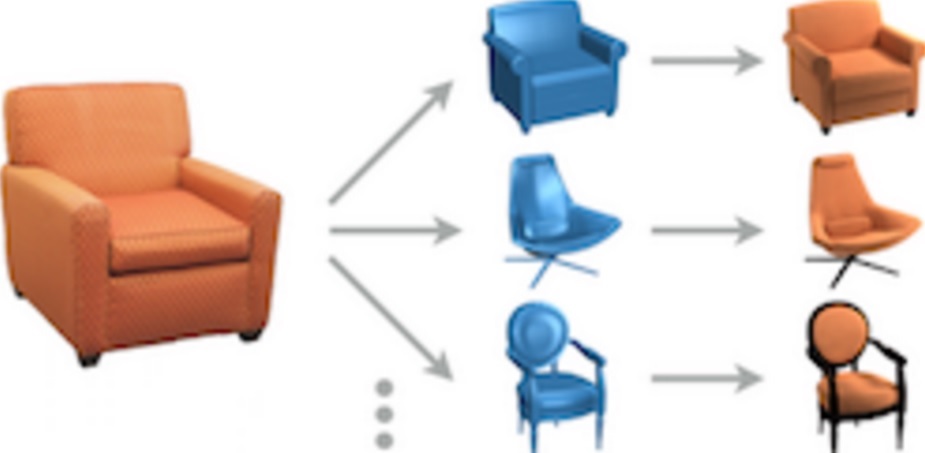 [SIGA16] Tuanfeng Wang, Hao Su, Qixing Huang, Jingwei Huang, Leonidas Guibas, and Niloy J. Mitra. Unsupervised Texture Transfer from Images to Model Collections. ACM Transaction on Graphics 35(6) (Proc. Siggraph Asia 2016).
|
|