Illumination Invariant Color Learning
Our generalized color learning enables the robot to model colors using a hybrid color representation thereby performing planned color learning both inside the lab and in less constrained settings outside the lab. In our previous work, we have also shown that we can enable the robot to recognize transitions between known illumination conditions, thereby achieving a certain degree of illumination invariance. We extend this approach significantly by enabling the robot to detect changes in illumination conditions autonomously, without any prior knowledge of the illumination conditions. The robot is also able to autonomously adapt to the changes in illumination conditions by planning a suitable motion sequence and learning the desired colors. Each illumination condition is characterized by a set of image distributions in the normalized RGB (r, g, b) color space. We also calculate the distances between each pair of image distributions corresponding to an illumination condition to arrive at the distribution of distances, which we model as a Gaussian. Using these the robot is able to detect significant changes in illumination.
Here, we provide a set of images showing the segmentation performance corresponding to different illumination conditions. When the robot learns a color map under a particular illumination condition and then the illumination condition is changed significantly, the robot's segmentation performance is very bad. But then the robot is able to detect the change in illumination and learn the required colors, and the segmentation performance is good once more.
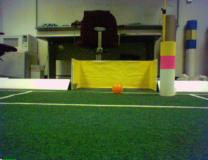
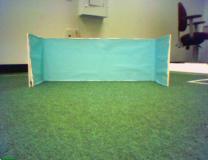
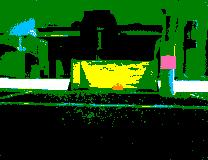
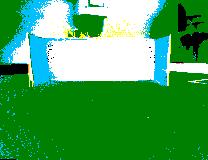
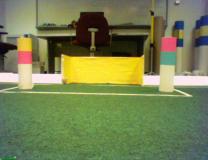
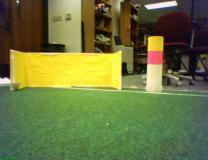
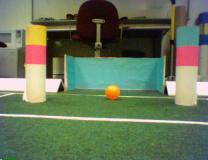
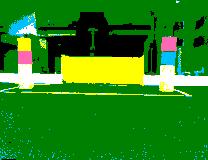
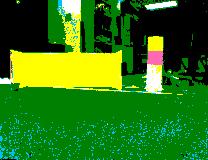
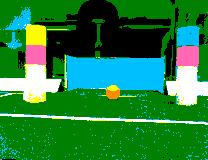
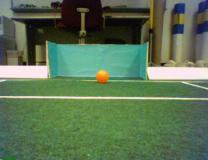
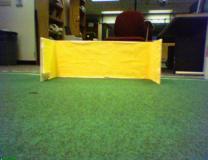
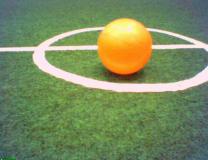
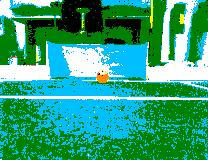
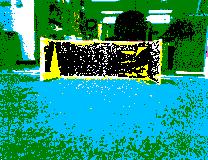
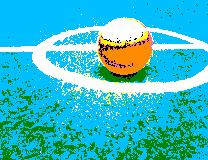
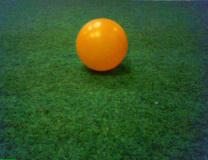
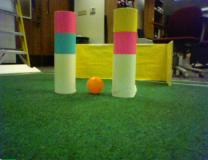
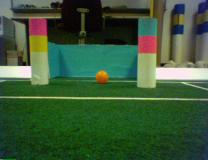
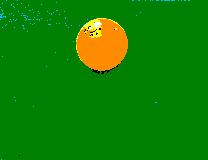
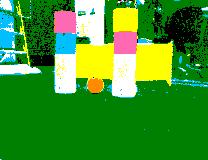
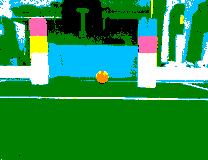
We also have some Images and videos corresponding to the color learning task and the planned color learning task.
Full details of our approach are available in the following paper:
- Color Learning on a Mobile Robot:
Towards Full Autonomy under Changing Illumination
Mohan Sridharan and Peter Stone.
The International Joint Conference on Artificial Intelligence (IJCAI), Hyderabad, India, January 2007. To Appear.