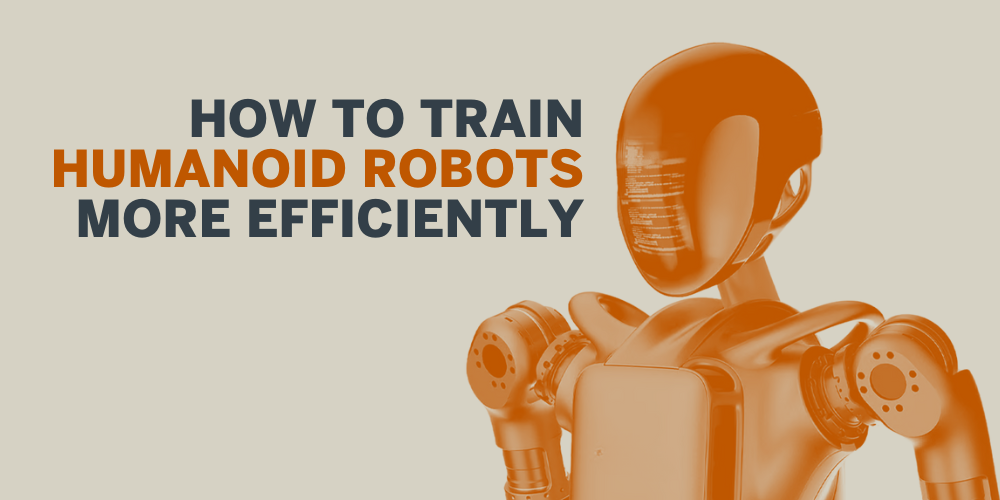
The Robot Perception and Learning Lab launched DexMimicGen, a new data generation system to improve training for humanoid robots. It builds on the lab’s earlier system, MimicGen, to predict humanoid autonomous robot movements from a small set of human demonstrations.
Traditionally, humans would need to perform hundreds or even thousands of demonstrations to generate the data needed to train autonomous humanoid robots. While MimicGen only applies to robots with limited dexterity, DexMimicGen can handle bots with two arms and five-fingered hands—enabling more advanced tasks.
“We don't want the human to have to repeatedly demonstrate a task and all its variations,” Kevin Lin, a first-year doctoral student and co-author of the paper, said. “The idea is that we just have a handful of demonstrations, maybe like three to five per task. Then, we use (DexMimicGen) to multiply these demonstrations to (a set of) different (object pose) variations.”
The team generated 21,000 training demos from just 60 human examples using devices like an Apple Vision Pro and iPhones to program while controlling a “digital twin.” The human controls the bot using these devices as the robot collects the data. Next, DexMimicGen uses the data and generates more from that single demonstration. The simulations, second-year Master’s student Yuqi Xie said, could reduce the amount of human effort needed to train autonomous robots.
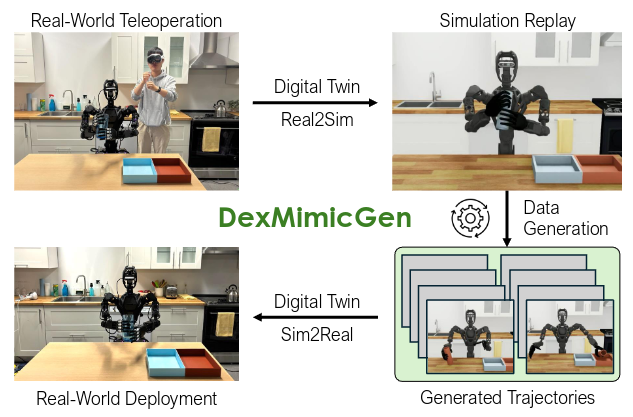
“If you want double the data, you need to double the human amount,” co-author Xie said. “If we just stick to the real data, there will be some collapse (at) some point, but if we can truly make use of all the simulation data, there's an actual infinite data source.”
Lin said the team hopes to continue working on the DexMimicGen for more widespread capacity. The researchers are now working to close the gap between simulation training and real-world performance.
“The rendering is different,” Lin said. “For certain tasks, certain differences between simulation and reality can be kind of ignored in some ways, but for other tasks the difference in physics between the real world and the simulator is noticeable.”
Additionally, researchers are working to improve the accuracy of robot-object interaction data while making the data generation as efficient as possible. The team noticed DexMimicGen’s accuracy tends to plateau around 80% for certain tasks, but simply scaling further could cost more computing power without improving on neural network policy training.
“The challenge with naively scaling up data is that it's going to be super expensive,” Lin said. “In terms of generating data, there is really a trade-off between coverage and plausibility.”
The demand for humanoid robots is dramatically increasing. The industry is predicted to reach $38 billion by 2035 with applications in healthcare, disaster rescue, manufacturing, and logistics. Ultimately, the team hopes to revolutionize how robots learn and make the process faster, cheaper, and more scalable.
“Maybe we don't need to rely on having people just collect data for super long hours,” Lin said. “We can just ask people to collect a few demonstrations, and then we automatically multiply the diversity of the data.”