Professor Kristen Grauman Elected Fellow of the American Association for the Advancement of Science
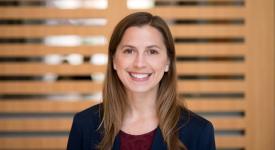
04/18/2024 - UT Computer Science Professor Kristen Grauman is among the eleven faculty members at The University of Texas at Austin who have been elected fellows of the American Association for the Advancement of Science (AAAS), including prominent figures in artificial intelligence from the university’s Department of